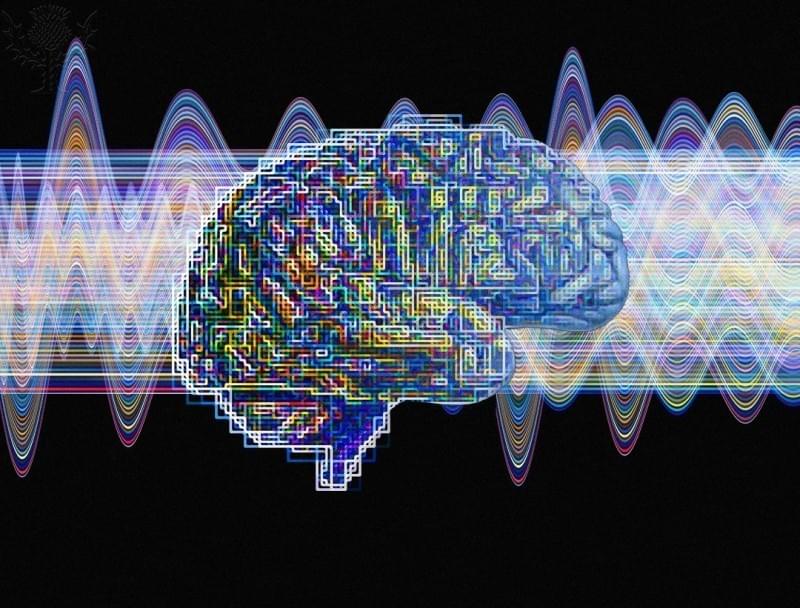
- The human brain is remarkably dynamic; we want to know why.
LIFESPAN NEURAL DYNAMICS GROUP
Research
Housed within the Max Planck UCL Centre for Computational Psychiatry and Ageing Research, the Lifespan Neural Dynamics Group (LNDG) seeks to understand how and why the human brain fluctuates so markedly from moment to moment. In particular, we examine brain signal variability and dynamics in relation to six core research foci (see below): lifespan development, cognition, neuromodulation, structural/functional connectivity, transcranial stimulation, and methods/modelling. Accordingly, we have an inherent multivariate focus that allows the examination of brain signal variability phenomena across multiple levels of analysis. The LNDG is graciously supported by the Max Planck UCL Centre, the Centre for Lifespan Psychology at the MPI for Human Development, and by an Emmy Noether grant from the German Research Foundation (DFG).
Lifespan Development and Aging
Degradation of neural dynamics with age
Early theory on aging, and in particular, the age-based degradation of cognition, is historically ascribed to some form of heightened “neural noise” that interferes with efficient processing (e.g., Crossman & Szafran, 1956; Cremer and Zeef, 1987; Welford, 1965, 1981). However, these early theories had rarely been tested by examining within-subject brain signals directly. Perhaps counterintuitively, our extant in vivo work consistently shows that older, poorer performers may generally exhibit less moment-to-moment brain signal variability than younger, higher performing adults (e.g., Garrett et al., 2010, 2011, JNeurosci; 2013 Cerebral Cortex; 2013 NBR; 2015 PNAS). Overall, our group seeks to understand why healthy, higher performing brains are highly and robustly dynamic across moments (adaptability, dynamic range, multi-stability?), and how this changes with development and aging.
Cognition
Behavioral relevance of neural dynamics
Our interests in the relations between cognition and brain signal variability have been broad to date, spanning simple and complex perceptual decision-making (e.g., Kloosterman et al., 2019, eLife), internal vs. externally modulated states (e.g., Grady and Garrett, 2018, NeuroImage), working (e.g., Garrett et al., 2015, PNAS) and long-term memory (Kloosterman et al., in prep), and reinforcement learning (Skowron et al., in prep) using a variety of stimulus types, cognitive performance metrics, and model parameterizations. Broadly, we find that better performers have higher brain signal variability, and that within-person signal variability can parametrically tune with changes in task difficulty (Garrett et al., 2013a,b, Cerebral Cortex; 2013, NBR; 2015 PNAS). We also continue to examine whether variability may be an expression of the flexible modulation of brain states that allows a probabilistic search for an optimal state, given either unexpected or anticipated changes in the environment (see Garrett et al., 2013, NBR).
Structural/Functional Connectivity
A basis for understanding local dynamics
It is well known that functional dynamics occur around a static anatomical (white matter) skeleton (Honey et al., 2009). Computational models suggest that realistic network dynamics, and the influence of noise within them, cannot exist without a healthy, biologically plausible WM structure (Deco et al., 2009). However, it remains unknown to what extent the WM skeleton constrains functional dynamics/variability in humans, and across the lifespan. Conversely, although structural connections form the “core skeleton” of brain function, functional connections also exist broadly in absence of direct structural connections (Deco et al., 2009; Ghosh et al., 2008; Honey et al., 2007). Notably, a theoretically and computationally informed contributing factor to brain signal variability effects is that our neural system can explore a broad repertoire of brain states/networks from moment to moment when variability is present (Deco et al., 2009; 2011; Garrett et al., 2013, NBR). Accordingly, a healthy, developed brain (i.e., in young adults) may be more flexible in the face of changing systemic or environmental demands, as it can reconfigure efficiently as required. Assuming that node-based signal variability reflects a dynamic system that is constantly reconfiguring in strength and direction, it is clear that explicitly measuring network-level dynamics is required to determine the bounds of node-based dynamics. We are examining these issues in detail within the LNDG. A recent attempt to link node-wise temporal dynamics to network-level effects (Garrett et al., 2018, NeuroImage) revealed that the vast majority of local temporal variability may be a reflection of network communication/integration rather than local “noise.” Crucially, the thalamus provided a unique and fundamental variability-based signature of how the brain integrates across scales, providing a key anatomical target for future LNDG work probing the generation and mechanisms of temporal variability in the brain.
Neuromodulation
Neurochemical bases for neural dynamics
We are interested in linking theories of dopamine (DA)-based neural “precision” (e.g., Ballo et al., 2012; Coull et al., 2012; Friston, 2010) and dynamics to brain signal variability effects. It is an interesting paradox that signal variability often appears functional for healthy neural systems, yet optimal DA function is presumed to generally invoke tighter bounds on temporal coding. We are currently collaborating with various research groups in Europe to investigate how DA agonists, antagonists, and PET-based DA binding potential may relate to working memory- and reinforcement-learning-based brain signal variability in young and older adults. We find that DA agonism via amphetamine increases BOLD variability in older adults (Garrett et al., 2015, PNAS; see fig above), DA antagonism decreases BOLD variability in younger adults (Garrett et al., in prep), and DA binding (using PET imaging) correlates with BOLD variability in a regionally-dependent manner (Guitart-Masip et al., 2016, Cerebral Cortex; Garrett et al., in prep). DA thus continues to be a primary neurochemical target within the LNDG, with our interests recently expanding to include the norepinephrine (e.g., systemic explore/exploit trade-offs) and glutamate/GABA systems (e.g., state-configuration via excitation/inhibition).
Transcranial Stimulation
Boosting deficient dynamics?
Our initial studies in this domain focus on directly invoking stochastic resonance, which is classically observed when the presence of additive noise allows input signals to be better detected in the brain. Historically, “noise” has been experimentally applied to the nervous system in a number of ways via noisy peripheral visual/tactile/auditory stimuli (e.g., Collins et al., 1996; Wells et al., 2005). However, good evidence for whether/how these peripherally applied “noise” sources reach the brain has been lacking. Alternatively, transcranial noise stimulation methods (tRNS) provide a more direct route to the cortex of the brain, thus piquing our interest in simultaneous tRNS-neuroimaging to examine links between stimulation and brain signal dynamics. Overall, we seek to use tRNS as a “causal” technique for restoring impoverished signal variability levels in older adults.
Methods/Modeling
Measures
We continue to both develop and apply brain signal variability methods, moving beyond general variance measures to ask more explicit questions about the nature of that variance (e.g., spectral content and structure, time delay embedding). We are also inherently interested in discrete patterns in brain signals (e.g., entropy), and how they relate to overall variance (*Grandy, *Garrett et al., 2016). For example, we continue to optimize the estimation of multi-scale entropy that effectively eliminates variance-based biases from computation (Kosciessa et al., in prep; Kloosterman et al., in prep), permitting entropy to be directly compared with oscillatory dynamics within-person (e.g., Kosciessa et al., 2019).
Statistical and computational modeling
We have a keen interest in multivariate models (e.g., singular value decomposition (SVD)-based techniques) that best enable various correlates of signal dynamics to be simultaneously examined. We are currently exploring how SVD models can be optimized within a mixed-modeling framework in an attempt to link latent-level parametric cognitive performance to parametric task-based brain dynamics (e.g., Garrett et al., 2013 Cereb Cortex; Garrett et al., 2015 PNAS). We also continue to develop whole-to-whole brain multivariate models linking different neuroimaging modalities (e.g., ASL and BOLD; dopamine PET and BOLD; EEG and BOLD; DWI and BOLD; e.g., Garrett et al., 2017, Sci Rep; *Burzynska, *Garrett, et al., 2013, JNeurosci).
We also utilize and develop various computational models of brain and behaviour. For example, we have recently leveraged the HMAX model of the ventral visual cortex to estimate how the complexity of visual input may drive neural variability during perception (Garrett et al., 2018). With regard to behaviour, we have also modelled within-person, reward-driven perceptual decision-making via hierarchical drift diffusion modelling (Kloosterman et al., 2019, eLife), and now deploy various reinforcement-learning models across a host of probabilistic reward-based paradigms.
Software Development
An important goal of our proposed work is to support the future examination of brain signal variability in neuroimaging through open-source software. At present, no analysis package provides for a comprehensive brain signal variability analysis. As such, we continue to develop an event-related and block-design fMRI-based Variability Toolbox (VarTbx) for SPM. Mimicking a Level-1 analysis, our toolbox allows SPM users (or more generally, Matlab users) to import any preprocessed fMRI data, choose from a number of different variability measures, and to output resulting individual maps for further analysis within SPM’s standard Level-2 module (or elsewhere). The first version of VarTbx is now available; see our software page for details. We further support open science by making all code/algorithms freely available on our LNDG Github repository (https://github.com/LNDG).
Team
The LNDG brain trust
Douglas D. Garrett, Ph.D.
Principal Investigator/Senior SCientist
Prior to heading the Lifespan Neural Dynamics Group (LNDG) within the Max Planck UCL Centre for Computational Psychiatry and Ageing Research, Doug completed degrees at the University of Victoria (BA) and University of Toronto (MA/Ph.D., 2011). He was also a Fellow with the MPS-UCL Initiative for Computational Psychiatry and Ageing Research (2011-2013), and a Research Scientist/Project Leader for the Intra-Person Neural Dynamics Project at the Center for Lifespan Psychology, Max Planck Institute for Human Development in Berlin, Germany. Outside of the lab, you’ll find Doug on a mountain, playing guitar or hockey, or running in-house studies with his twin boys :-) CV Google Scholar X Bluesky
Liliana Polanski
Postdoctoral fellow
Liliana joined the LNDG lab as a research assistant in 2017, and completed her PhD in 2024. She’ received a BSc degree in Psychology from Humboldt University and is currently doing her master’s in Social, Cognitive and Affective Neuroscience at Freie Unversity Berlin. Liliana is interested in brain network dynamics and decision making, especially with regard to understanding the exploration-exploitation dilemma.
Alexander Skowron
Postdoctoral fellow
Alex joined the lab in 2017 as an intern/master's student, and completed his PhD in 2024. He obtained his Bachelor’s degree in Psychology from Leiden University and his master's in the Social, Cognitive and Affective Neuroscience Master's program at Freie Universität Berlin. Alex is particularly interested in multimodal brain-signal variability and entropy metrics in the context of learning and memory. In his free time, he enjoys participating in Uni sports and playing video games.
Fabian Kamp
ph.d. student
Fabian finished his MSc in the Social, cognitive and affective Neuroscience (MSc) at the FU, after joined the LNDG in January 2020. He recently re-joined the LNDG as an incoming PhD student in Oct 2022. He is particularly interested in computational psychiatry, brain simulations, and neuroplasticity. In his free time, Fabian enjoys traveling - especially to Italy.
Zoya Mooraj
Ph.d. student
Zoya joined the LNDG in November 2022 as a doctoral student in the IMPRS COMP2PSYCH program after completing her masters at the Berlin School of Mind and Brain at Humboldt-Universität zu Berlin. She is particularly interested in metacognition and variability across the lifespan, particularly in aging. In her free time, she enjoys reading, inline skating, and thinking about the perception of time.
Primary LNDG Collaborators
Lars Bäckman (Aging Research Center, Karolinska Institute)
Cheryl Grady (Rotman Research Institute, Baycrest; University of Toronto)
Marc Guitart-Masip (Aging Research Center, Karolinska Institute)
Ulman Lindenberger (MPI for Human Development)
Stu MacDonald (University of Victoria)
Lars Nyberg (Umeå University)
Thad Polk (University of Michigan)
Naftali Raz (Wayne State University)
Alireza Salami (Aging Research Center, Karolinska Institute)
Greg Samanez-Larkin (Duke)
LNDG Alumni
Dag Alnæs (scientist)
Aidan Brinda (intern)
Vivien Chopurian (research assistant)
Cristiana Dimulescu (master's student)
Samira Epp (master's student)
Elisabeth Höhne (intern)
Matthias Kerkemeyer (intern)
Niels Kloosterman (postdoctoral fellow)
Julian Kosciessa (PhD student and postdoctoral fellow)
Lanfang Liu (visiting Ph.D. student)
Kristoffer Månsson (postdoc)
Janina Marchner (research assistant)
Alistair Perry (postdoctoral fellow)
Claire Pleche (master's student)
Sarah Polk (research assistant)
José Yordan Ramirez (research assistant)
Neslihan Sener (master's student)
Stefan Schmidt (programmer)
Marta Stojanovic (master's student)
Alireza Tarikhi (research assistant)
Laura Terlau (master's student)
Marija Tochasde (master's student)
Evi van den Elzen (master's student)
Leo Waschke (postdoctoral fellow)
Patrick Werner (research assistant
Iris Wiegand (postdoctoral fellow)
Steffen Wiegert (lab manager)
Publications
Our work
Manuscripts under review
Skowron, A., Kosciessa, J.Q., Lorenz, R., Hertwig, R., van den Bos, W., & Garrett, D.D. (under review). Neural variability compresses with increasing belief precision during Bayesian inference. bioRxiv. Link
Garrett, D.D., Kloosterman, Epp, S., Chopurian, V., Kosciessa, J.Q., Waschke, L., Skowron, A., Shine, J.M., Perry, A., Salami, A., Rieckmann, A., Papenberg, G., Wåhlin, A., Karalija, N., Andersson, M., Riklund, K., Lövdén, M., Bäckman, L., Nyberg, L., & Lindenberger, U. (under review). Dynamic regulation of neural variability during working memory reflects dopamine, functional integration, and decision-making. bioRxiv. Link
Peer reviewed articles
Haupt, M., Garrett, D.D., & Cichy, R. (in press). Healthy aging delays and differentiates high-level visual representations. Current Biology. Link
Tsikonofilos, K., Kumar, A., Ampatzis,, K., Garrett, D.D., Månsson, K.N.T. (in press). The promise of investigating neural variability in psychiatric disorders. Biological Psychiatry. Link
Lalwani, P., Polk, T., & Garrett, D.D. (2025). Modulation of neural variability: Age-related reduction, GABAergic basis, and behavioral implications. eLife, 14: e83865. Link
Mooraj, Z., Salami, A., Campbell, K.L., Dahl, M.J., Kosciessa, J.Q., Nassar, M.R., Werkle-Bergner, M., Craik, F.I.M., Lindenberger, U., Mayr, U., Rajah, M.N., Raz, N., Nyberg, L., & Garrett, D.D. (2025). Towards a functional future for the cognitive neuroscience of human aging. Neuron, 113, 154-183. Link
*Waschke, L., *Kamp, F., van den Elzen, E., Krishna, S., Lindenberger, U., Rutishauser, U., & Garrett, D.D. (2025). Single-neuron spiking variability in hippocampus dynamically tracks sensory content during memory formation in humans. Nature Communications, 16:236. Link
Papenberg, G., Karalija, N., Salami, A., Johansson, J., Wåhlin, A., Andersson, M., Axelsson, J., Garrett, D.D., Riklund, N., Lindenberger, U., Lövdén, M., Nyberg, L., & Bäckman, L. (2025). Aging-related losses in fronto-striatal dopamine D2 receptor availability are linked to working-memory decline across five years. Cerebral Cortex, bhae481. Link
Kosciessa, J., Mayr, U., Lindenberger, U., & Garrett, D.D. (2024). Broadscale dampening of uncertainty adjustment in the aging brain. Nature Communications, 15:10717. Link
Shine, J.M., Lewis, L.D., Garrett, D.D., & Hwang, K. (2023). The impact of the human thalamus on brain-wide information processing. Nature Reviews Neuroscience. Link
Krohn, S., von Schwanenflug, N., Waschke, L., Romanello, A., Gell, M., Garrett, D.D., & Finke, C. (2023). A spatiotemporal complexity architecture of human brain activity. Science Advances, 9, eabq385. Link
Nyberg, L., Karalija, N., Papenberg, G., Salami, A., Andersson, M., Pedersen, R., Vikner, T., Garrett, D.D., Riklund, N., Wåhlin, A., Lövdén, M., Lindenberger, U., & Bäckman, L. (2022). Longitudinal stability in working memory and frontal activity reflect general brain maintenance. Scientific Reports, 12, 20957. Link
Månsson, K.N.T., Waschke, L., Manzourid, A., Furmark, T., Fischer, H., & Garrett, D.D. (2022). Moment-to-moment brain signal variability reliably predicts psychiatric treatment outcome. Biological Psychiatry, 91, 658-666. Link
Waschke, L., Donoghue, T., Fiedler, L., Smith, S., Garrett, D.D., Voytek, B., & Obleser, J. (2021). Modality-specific tracking of attention and sensory statistics in the human electrophysiological spectral exponent. eLife, 0:e70068. Link
Lalwani, P., Garrett, D.D., & Polk, T. (2021). Dynamic recovery: GABA agonism restores neural variability in older, poorer performing adults. Journal of Neuroscience, 41, 9350-9360. Link
Korkki, S.,M., Papenberg, G., Karalija, N., Garrett, D.D., Riklund, N., Lövdén, M., Lindenberger, U., Nyberg, L., & Bäckman, L. (2021). Fronto-striatal dopamine D2 receptor availability is associated with cognitive variability in older individuals with low dopamine integrity. Scientific Reports, 11, 21089. Link
Garrett, D.D., Skowron, A., Wiegert, S., Adolf, J., Dahle, C.L., Lindenberger, U., & Raz, N. (2021). Lost dynamics and the dynamics of loss: Longitudinal compression of brain signal variability is coupled with declines in functional integration and cognitive performance. Cerebral Cortex, 31, 5239–5252. Link
Kosciessa, J.Q., Lindenberger, U., & Garrett, D.D. (2021). Thalamocortical excitability adjustments guide human perception under uncertainty. Nature Communications, 12:2430. Link
Waschke, L., Kloosterman, N., *Obleser, J., & *Garrett, D.D. (2021). Behaviour needs neural variability. Neuron, 109, 1-16. Link
Kloosterman, N.A., Kosciessa, J.Q., Lindenberger, U., Fahrenfort, J.J., & Garrett, D.D. (2020). Boosts in brain signal variability track liberal shifts in decision bias. eLife, 9:e54201. Link
Grady, C.L., Nichol, D., Rieck, J., & Garrett, D.D. (2020). Whole brain functional connectivity and representational similarity within the Face Network contribute to reduced discrimination of degraded faces in young and older adults. Cerebral Cortex, 10.1093/cercor/bhaa179. Link
Kosciessa, J.Q., Kloosterman, N.A., & Garrett, D.D. (2020). Standard multiscale entropy reflects neural dynamics at mismatched temporal scales: What’s signal irregularity got to do with it? PLOS Computational Biology: 16(5): e1007885. Link
Garrett, D.D., Epp, S.M., Kleemeyer, M., Lindenberger, U., and Polk, T.A. (2020). Higher performing older adults upregulate brain signal variability in response to feature-rich sensory input. NeuroImage. Link
Kosciessa, J.Q., Grandy, T.H., Garrett, D.D., & Werkle-Bergner, M, (2020). Single-trial characterization of neural rhythms: potential and challenges. NeuroImage. Link
Liu, L., Zhang, Y., Zhou, Q., Garrett, D.D. Lu, C., Chen, A., Qiu, J., Ding, G. (2020). Auditory-Articulatory Neural Coupling between Listener and Speaker during Verbal Communication. Cerebral Cortex. doi: 10.1093/cercor/bhz138. Link
Kloosterman, N.A., de Gee, J.W., Werkle-Bergner, M., Lindenberger, U., *Garrett, D.D., *Fahrenfort, J.J. (2019). Humans strategically shift decision bias by flexibly adjusting sensory evidence accumulation. eLife, 8:e37321. Link
Salami, A., Garrett, D.D., Rieckmann, A., Wåhlin, A., Papenberg, G., Karalija, N., Andersson, M., Axelsson, J.,Johansson, J., Riklund, K., Lövdén, M., Lindenberger, U., Bäckman, L., & Nyberg, L. (2019). Dopamine D2 binding potential modulates neural signatures of working memory in a load-dependent fashion. Journal of Neuroscience, 39, 537-547. Link
Garrett, D.D., Epp, S., Perry, A., & Lindenberger, U. (2018). Local variability reflects lower dimensional functional integration in the human brain. NeuroImage, 183, 776-787. Link preprint
Salami, A., Rieckmann, A., Karalija, N., Avelar-Pereira, B., Andersson, M., Wåhlin, A., Papenberg, G., Garrett, D.D., Riklund, K., Lövdén, M., Lindenberger, U., Bäckman, L., & Nyberg, L. (2018). Neurocognitive profiles of healthy older adults with working-memory dysfunction. Cerebral Cortex, 28, 2525–2539. Link
Grady, C.L. & Garrett, D.D. (2018). Brain Signal Variability is Modulated as a Function of Internal and External Demand in Younger and Older Adults. NeuroImage, 169, 510-523. Link
Halliday, D., Mulligan, B., Garrett, D.D., Schmidt, S., Hundza, S., Garcia-Barrera, M., Stawski, R., MacDonald, S.W.S. (2018). Mean and Variability In Functional Brain Activations Differentially Predict Executive Function in Older Adults: A Novel Investigation Employing Functional Near Infrared Spectroscopy. Neurophotonics, 5, 011013, doi: 10.1117/1.NPh.5.1.011013. Link
Lövdén, M., Karalija, N., Andersson, M., Wåhlin, A., Axelsson, J., Köhncke, Y., Jonasson, L.S., Rieckmann, A., Papenberg, G., Garrett, D.D., Guitart-Masip, M., Salami, A., Riklund, K., Bäckman, L., Nyberg, L., & Lindenberger, U. ( 2017). Latent-Profile Analysis Reveals Behavioral and Brain Correlates of Dopamine-Cognition Associations. Cerebral Cortex, doi.org/10.1093/cercor/bhx253. Link
Garrett, D.D., Lindenberger, U., Hoge, R., and Gauthier, C.J. (2017). Age differences in brain signal variability are robust to multiple vascular controls. Scientific Reports, 7, 10149. LinkNyberg, L., Karaljia, N., Salami, A., Andersson, M., Wåhlin, A., Kaboovand, N., Axelsson, J., Rieckmann, A., Papenberg, G., Garrett, D.D., Ricklund, K., Lövden, M., Lindeberger, U., Köhncke, Y., and Bäckman, L. (2016). Dopamine D2 receptor availability is linked to striato-hippocampal functional connectivity and episodic memory. Proceedings of the National Academy of Sciences of the United States of America, 113, 7918-7923. Link
*Grandy, T., *Garrett, D.D., Schmiedek, F., and Werkle-Bergner, M. (2016). On the estimation of brain signal entropy from sparse neuroimaging data. Scientific Reports, 6, 23073. Link
Guitart-Masip, M., Salemi, A., Garrett, D.D., Rieckmann, A., Lindenberger, U., & Bäckman, L. (2016). BOLD variability is related to dopaminergic transmission and cognitive aging. Cerebral Cortex, 26, 2074–2083 . Link
Garrett, D.D., Nagel, I.E., Preuschhof, C., Burzynska, A.Z., Marchner, J., Wiegert, S., Jungehülsing, G., Nyberg, L., Villringer, A., Li, S-C., Heekeren, H.E., Bäckman, L., & Lindenberger, U. (2015). Amphetamine modulates brain signal variability and working memory in younger and older adults. Proceedings of the National Academy of Sciences of the United States of America, 112, 7593-7598. Link
Garrett, D.D., McIntosh, A.R., & Grady, C.L. (2014). Brain signal variability is parametrically modifiable. Cerebral Cortex, 24, 2931-2940. Link
Grady, C.L. & Garrett, D.D. (2013). Understanding variability in the BOLD signal and why it matters for aging. Brain Imaging and Behavior, doi: 10.1007/s11682-013-9253-0. Link
*Burzynska, A.Z., *Garrett, D.D., Preuschhof, C., Nagel, I.E., Li, S-C., Bäckman, L., Heekeren, H.E., & Lindenberger, U. (2013). A scaffold for efficiency in the human brain. Journal of Neuroscience, 33, 17150-17159. Link
Garrett, D.D., Samanez-Larkin, G.L., MacDonald, S.W.S., Lindenberger, U., McIntosh, A.R., & Grady, C.L. (2013). Moment-to-moment brain signal variability: A next frontier in brain mapping? Neuroscience & Biobehavioral Reviews, 37, 610-624. Link
Garrett, D.D., Kovacevic, N., McIntosh, A.R., & Grady, C.L. (2013). The modulation of BOLD variability between cognitive states varies by age and processing speed. Cerebral Cortex, 23, 684-693. Link
Garrett, D.D., MacDonald, S.W.S., & Craik, F.I.M. (2012). Intraindividual reaction time variability is malleable: Feedback- and cognitive reserve-related reductions in variability with age. Frontiers in Human Neuroscience, 6, 101. doi: 10.3389/fnhum.2012.00101. Link
Garrett, D.D., Kovacevic, N., McIntosh, A.R., & Grady, C.L. (2011). The importance of being variable. Journal of Neuroscience, 31, 4496-4503. Link
Garrett, D.D., Kovacevic, N., McIntosh, A.R. & Grady, C.L. (2010). Blood oxygen level-dependent signal variability is more than just noise. Journal of Neuroscience, 30, 4914-4921. Link
Garrett, D.D., Grady, C.L., & Hasher, L. (2010). Everyday memory compensation: The impact of cognitive reserve, subjective memory, and stress. Psychology and Aging, 25, 74-83. Link
McIntosh, A.R., Kovacevic, N., Lippe, S, Garrett, D.D., Grady, C.L., & Jirsa, V. (2010). The development of a noisy brain. Archives of Italian Biology, 148, 323-337. Link
Lindstrom-Forneri, W., Tuokko, H., Garrett, D.D., & Molnar, F. (2010). Everyday competence: Theories, measurement strategies, and its relations to driving. Clinical Gerontologist, 33, 283-297. Link
Garrett, D.D., Tuokko, H., & Stajduhar, K. (2008). Planning for end-of-life care: Findings from the Canadian Study of Health and Aging. Canadian Journal on Aging, 27, 11-21. Link
Stepaniuk, J.A., Tuokko, H.A., McGee, P., Garrett, D.D. & Benner, E.L. (2008). Impact of transit training and free bus pass on public transportation use by older drivers. Preventative Medicine, 47, 335-337. Link
Dixon, R.A., Garrett, D.D., Lentz, T.L., Strauss, E., & Hultsch, D.F. (2007). Neurocognitive markers of mild cognitive impairment: Exploring the roles of speed and inconsistency. Neuropsychology, 21, 381-399. Link
Tuokko, H., Garrett, D.D., McDowell, I., Silverberg, N., & Kristjansson, B. (2003). Cognitive decline in high-functioning older adults: Reserve or ascertainment bias? Aging and Mental Health, 7, 259-270. Link
Chapters and Commentaries
Garrett, D.D., McIntosh, A.R., & Grady, C.L. (2011). Moment-to-moment brain signal variability can inform models of stochastic facilitation now. Nature Reviews Neuroscience, 12, 612. Link
Garrett, D.D., Kovacevic, N., McIntosh, A.R. & Grady, C.L., (2010). All within-subject BOLD standard deviations are not created equal [response to Mohr, P.N. & Nagel, I.E.]. Journal of Neuroscience, 30, 7755-7757. [doi:10.1523/JNEUROSCI.1560-10.2010]. Link
Dixon, R.A., Garrett, D.D., & Backman, L. (2008). Compensation in cognitive neurorehabilitation. In D.T. Stuss, G. Winocur and I.H. Robertson (Eds.), Cognitive Neurorehabilitation, 2nd Edition. Cambridge: Cambridge University Press. Link
Other contributions
Garrett, D.D. (2018). If you want to be a scientist parent, come to Germany. eLife. Link
Garrett, D.D. & Grady, C.L. (2010). Review of Stephens et al. (2010). Faculty of 1000, Dec, 2010.
Garrett, D.D. & Grady, C.L. (2010). Review of Gazzaniga (2010). Faculty of 1000, Aug, 2010.
Software
Openly available tools and algorithms developed by LNDG
Variability Toolbox
for SPM
(VarTbx)
VarTbx measures within-voxel time series variability in fMRI data. The VarTbx structure is intended to be similar to a standard SPM first-level analysis. You can then proceed to pass those first-level, variability-based images to a level-2 SPM analysis in order to model group effects of interest. However, the first-level NIFTI output files could also be used within other statistics programs of your choice (e.g., FSL, AFNI, PLS).
VarTbx currently supports modeling block designs with a boxcar model, and computes temporal variability using measures such as: detrended variance (VAR), detrended standard deviation (SD), mean squared successive difference (MSSD), and SQRT(MSSD). We plan to add additional modeling approaches and variability measures in future releases.
Using VarTbx is relatively straightforward. You first specify your first-level SPM model, as usual. You then save the model, which is used as input to specify all sessions, conditions, onsets, durations, and nuisance regressors for VarTbx. Finally, after choosing a variability metric of interest, variability-based NIFTI images are then produced. Click on the video above for a short VarTbx tutorial.
Extended
Better Oscillation
Detection (eBOSC)
The average power of rhythmic neural responses as captured by MEG/EEG/LFP recordings is a prevalent index of human brain function. Increasing evidence questions the utility of trial-/group averaged power estimates however, as seemingly sustained activity patterns may be brought about by time-varying transient signals in each single trial. Hence, it is crucial to accurately describe the duration and power of rhythmic and arrhythmic neural responses on the single trial-level.
In a recently published paper (Kosciessa et al., 2019, NeuroImage), we extended an existing rhythm detection algorithm (extended Better OSCillation detection: “eBOSC”) to systematically investigate boundary conditions for estimating neural rhythms at the single-trial level.
The eBOSC package is now freely available on Github.
Modified
multiscale entropy (mMSE)
Recently, we have developed a novel algorithm based on multiscale entropy (Costa et al. 2002) called modified multiscale entropy (mMSE) that directly quantifies the temporal irregularity of time-domain EEG/MEG/LFP signals at longer and shorter timescales. In general, patterns of fluctuations in brain activity that tend to repeat over time are assigned lower entropy, whereas more irregular, non-repeating patterns yield higher entropy. To allow the investigation of dynamic changes in signal irregularity, we developed mMSE as a time-resolved variant, while also permitting assessment of entropy over atypically longer time scales by calculating across discontinuous, concatenated segments.
For further details, see:
Kloosterman, N.A., Kosciessa, J.Q., Lindenberger, U., Fahrenfort, J.J., & Garrett, D.D. (2020). Boosts in brain signal variability track liberal shifts in decision bias. eLife, 9:e54201. Link
Kosciessa JQ, Kloosterman NA, Garrett DD (2020) Standard multiscale entropy reflects neural dynamics at mismatched temporal scales: What’s signal irregularity got to do with it? PLOS Computational Biology: 16(5): e1007885. Link
And for a FieldTrip tutorial and implementation, click here
Equity, Diversity, and Inclusion (EDI)
How we're built
The LNDG is built on diversity. Our work is a product of scientists of different professional backgrounds (mathematics, experimental and clinical psychology, statistics, computer science), genders, and working styles from 13 countries around the world. Through flexible workflows and adaptive policies, we work to promote and provide an inclusive, family-friendly working environment. For an excellent list of EDI resources relevant for team building and science/work, head here.
Contact the Lifespan Neural Dynamics Group
We welcome any questions or comments you have. Please contact us at:
Max Planck UCL Centre for Computational Psychiatry and Ageing ResearchBerlin
Max Planck Institute for Human Development
Lentzeallee 94
14195 Berlin, GermanyEmail: lndg@mpib-berlin.mpg.de
© 2025